Comprehensive Application of AI Fire Detection and Warning Technology in the Field of Electric Vehicle Charging
Introduction
This article delves into the innovative application of artificial intelligence (AI) technology in enhancing the safety of electric vehicle (EV) charging, particularly focusing on AI-based fire detection and warning systems. It elaborates on how high-precision sensors monitor critical parameters during the charging process, such as temperature, current, and voltage. AI technology can accurately detect potential fire hazards and promptly implement corresponding emergency actions. Furthermore, AI relies on big data analysis to continuously improve its fire detection capabilities and integrates with various intelligent systems to effectively elevate overall safety management.
Importance of AI Technology
AI technology plays a crucial role in precisely monitoring and predicting the state of electric bicycle batteries. It helps in early detection of abnormal conditions and provides users with personalized charging recommendations, thereby reducing risks associated with improper charging operations.
Applications of AI Technology
- Real-Time Monitoring and Warning: AI systems continuously collect data on battery voltage, current, temperature, etc., and use learning algorithms to detect overheating or other anomalies. Upon identifying potential risks, the system immediately alerts users or monitoring centers.
- Fault Diagnosis and Prediction: Adaptive neural network algorithms can identify trends in battery health changes and predict potential faults, such as accelerated aging or capacity decline.
- Electric Power Quality Analysis: AI analyzes electric power quality during the charging process to identify unstable power sources or grid fluctuations that could damage batteries.
- Intelligent Scheduling and Emergency Response: In emergencies, AI assists in decision-making by providing optimal emergency response plans, such as quickly dispatching nearby rescue teams.
Implementation Pathways
To ensure safety during the charging process, the application of AI technology involves:
- Data Collection: Using IoT technology to gather real-time operational data from electric bicycle batteries.
- Data Analysis: Employing adaptive neural network algorithms to analyze collected data and identify patterns that may lead to failures.
- Warning Mechanism: Establishing a warning mechanism based on analysis results to instantly alert when potential risks are detected.
- User Interaction: Developing applications or platforms for users to receive warnings and obtain safe charging guidelines.
- Remote Monitoring: Implementing cloud services for remote monitoring of battery status, providing data support for manufacturers and service providers.
Role of Adaptive Neural Network Algorithms
Adaptive neural network algorithms dynamically learn from large historical datasets, capturing patterns in battery state changes. They update models in real-time to maintain high prediction accuracy even as battery performance changes. These algorithms excel at handling complex relationships and interactions among multiple variables, which is essential for understanding the intricate chemical and physical processes within batteries.
Electric Power Quality and Battery Fire Cause Analysis
AI technology monitors electric power quality indicators during charging to prevent battery damage or failure caused by unstable power sources or grid fluctuations. Additionally, it analyzes historical data from battery fire incidents to identify common causes, such as overcharging, short circuits, or internal defects, optimizing charging strategies to prevent fires.
Future Prospects
Looking ahead, more intelligent solutions will be applied to electric bicycle safety management, including:
- Personalized Charging Strategies: Based on user habits and actual vehicle conditions, AI systems generate tailored charging strategies to extend battery life.
- Battery Health Management: Regular health checks allow AI to predict remaining battery life and provide replacement or maintenance recommendations.
- Environmental Adaptability Analysis: Considering external factors like temperature and humidity, AI offers comprehensive usage advice to users.
In summary, the application of AI technology not only enhances the safety of electric bicycle charging but also brings new transformation opportunities to the entire electric bicycle industry.
Detailed Content Translation
Abstract
This paper deeply analyzes the innovative application of artificial intelligence technology in the field of electric vehicle (EV) safety charging, especially the comprehensive promotion of AI-based fire detection and warning technology in EV charging. The article details how high-precision sensors monitor key parameters during the charging process, including temperature, current, and voltage, enabling AI to accurately identify potential fire risks and swiftly take appropriate emergency measures. Furthermore, AI technology leverages big data analysis to continuously optimize fire detection capabilities and collaborates with various intelligent systems, effectively improving overall safety management. The paper emphasizes the importance of AI technology in monitoring and predicting the state of electric bicycle batteries, enhancing the safety of the charging process through real-time monitoring warnings, fault diagnosis and prediction, electric power quality analysis, and intelligent scheduling and emergency response. Finally, the paper looks forward to future applications of AI technology in electric bicycle safety management, including personalized charging strategies, battery health management, and environmental adaptability analysis, bringing new opportunities for transformation in the electric bicycle industry.
Background
With the growing popularity of electric vehicles and increasing charging demands, the comprehensive application of AI fire detection and warning technology in EV charging has become increasingly important. Utilizing AI technology can achieve real-time monitoring and early warning of potential fire hazards during the charging process, ensuring the safe operation of charging facilities and user safety.
Specific Implementation
AI fire detection and warning technology uses high-precision sensors installed at charging stations to monitor real-time parameters such as temperature, current, and voltage during the charging process. These sensors capture minor changes and, through advanced data analysis algorithms, identify potential fire risks. Once an anomaly is detected, the system immediately issues an alarm and takes corresponding emergency measures, such as automatically cutting off power or activating fire suppression devices to prevent fires.
Moreover, AI fire detection and warning technology can enhance its detection capabilities through big data analysis. By analyzing historical data, the system learns and identifies more fire risk patterns, improving the accuracy and timeliness of warnings. Simultaneously, this technology can collaborate with other intelligent systems, such as fire protection and surveillance systems, sharing data and working together to further enhance overall safety management.
Key Technologies
To ensure the safety of electric bicycles during charging, the application of AI technology mainly involves several steps:
- Data Collection: Using IoT technology to collect real-time operational data from electric bicycle batteries, including but not limited to temperature, current, and voltage information.
- Data Analysis: Through adaptive neural network algorithms, performing in-depth analysis on the collected data to identify abnormal patterns that could lead to failures.
- Warning Mechanism: Based on analysis results, establishing a warning mechanism to instantly issue alerts when potential risks are detected.
- User Interaction: Developing corresponding applications or platforms so users can receive warning information and obtain guidelines for safe charging operations.
- Remote Monitoring: Implementing cloud services to enable remote monitoring of battery status, providing data support for manufacturers and service providers, facilitating better after-sales service and technical support.
Role of Adaptive Neural Network Algorithms
Adaptive neural network algorithms are machine learning methods that can self-adjust over time. They can automatically adjust weights and structures based on different input data to improve prediction accuracy. In the electric bicycle charging accident detection and warning system, adaptive neural network algorithms can:
- Dynamic Learning: Learn from large historical datasets to capture patterns in battery state changes, maintaining high prediction accuracy even as batteries age or environmental conditions change.
- Real-Time Updates: Continuously update models with new data, ensuring they remain in optimal condition to address any changes in battery performance.
- Complex Relationship Modeling: Handle nonlinear relationships and interactions among multiple variables, which is crucial for understanding the complex chemical and physical processes within batteries.
Analysis of Electric Power Quality and Battery Fire Causes
During the charging process of electric bicycles, electric power quality directly affects battery lifespan and safety. AI technology can monitor indicators such as grid frequency and voltage fluctuations to timely detect unstable power sources or grid fluctuations, preventing battery damage or failure.
Additionally, AI technology can analyze historical data from battery fire incidents to identify common causes, such as overcharging, battery short circuits, or internal defects. Through these analyses, charging strategies can be optimized to prevent fire accidents.
Future Outlook
As AI technology continues to evolve, we can foresee more intelligent solutions being applied to electric bicycle safety management. Beyond the mentioned real-time monitoring and warning systems, future applications include:
- Personalized Charging Strategies: Based on user habits and vehicle conditions, AI systems can generate personalized charging strategies to help users extend battery life.
- Battery Health Management: Through regular health checks, AI can predict battery remaining life and provide replacement or maintenance suggestions.
- Environmental Adaptability Analysis: Considering external factors like temperature and humidity, AI can offer comprehensive usage advice to users.
In conclusion, the application of AI technology not only improves the safety of electric bicycle charging but also brings new transformation opportunities to the entire electric bicycle industry.
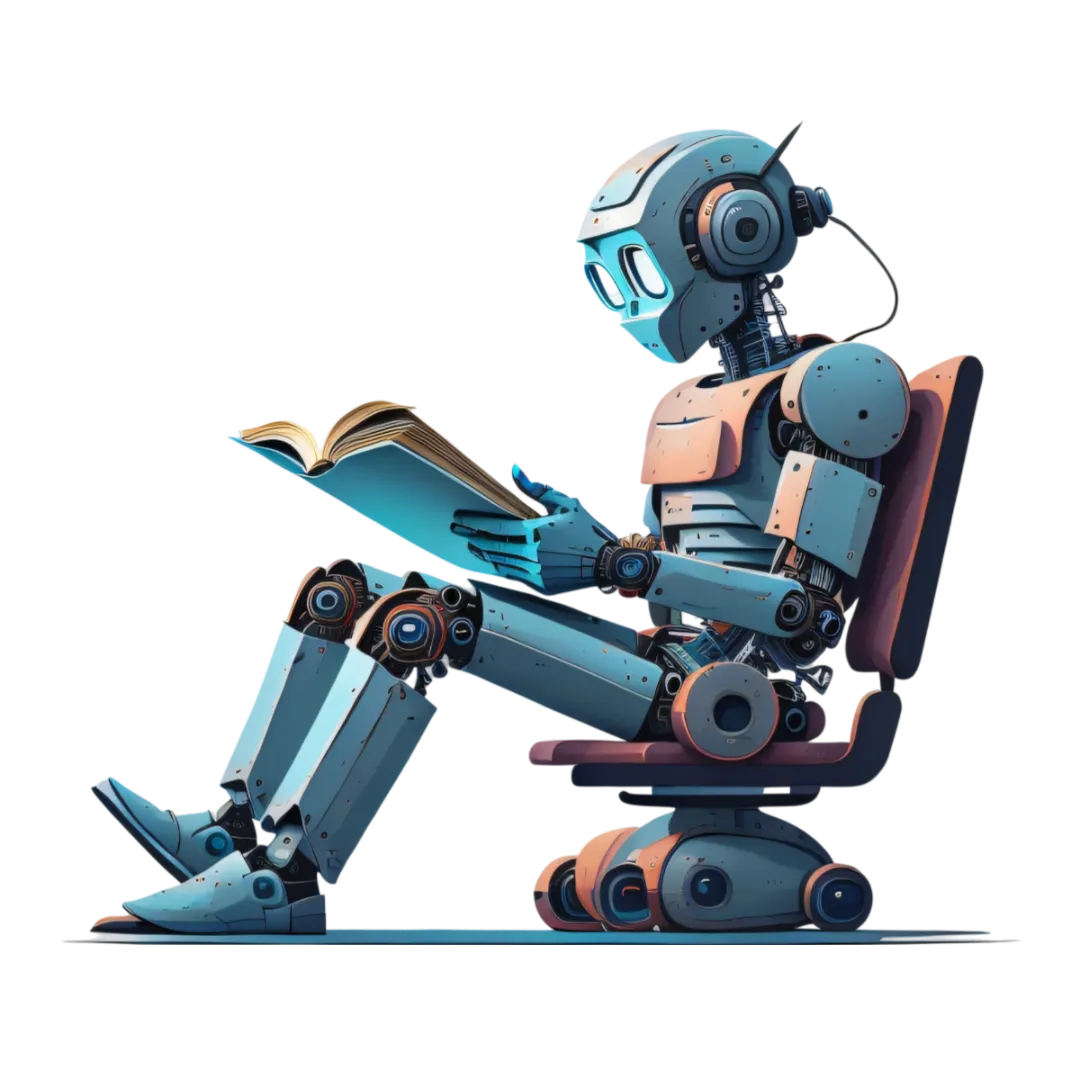
安充宝AI火灾检测预警技术在电动车充电领域的全面应用
全文概要:本文深入剖析了人工智能技术在电动车安全充电领域的创新应用,特别是人工智能火灾检测预警技术在电动车充电领域的全面推广。文章详细阐述了通过高精度传感器对充电过程中的关键参数,包括温度、电流、电压等进行实时监测,人工智能技术能够精准识别潜在的火灾风险,并迅速采取相应的应急措施。进一步地,人工智能技术依托大数据分析,不断优化火灾检测能力,并与各类智能系统实现联动,从而有效提升整体的安全管理水平。文章还强调了AI技术在电动自行车电池状态监测和预测中的重要性,能够通过实时监测预警、故障诊断与预测、电能质量分析和智能调度与应急响应等应用场景,提高充电过程的安全性。自适应神经网络算法在其中扮演了关键角色,能够动态学习、实时更新和处理复杂关系建模。最后,文章展望了AI技术在电动自行车安全管理中的未来应用,包括个性化充电策略、电池健康管理以及环境适应性分析等,这些智能解决方案将为电动自行车产业带来新的变革机遇。
随着电动车的普及和充电需求的增加,AI火灾检测预警技术在电动车充电领域的全面应用显得尤为重要。通过利用人工智能技术,可以实现对充电过程中可能出现的火灾隐患进行实时监控和早期预警,从而确保充电设施的安全运行和用户的使用安全。
具体来说,AI火灾检测预警技术通过安装在充电站的高精度传感器,实时监测充电过程中的温度、电流、电压等关键参数。这些传感器能够捕捉到微小的变化,并通过先进的数据分析算法,识别出潜在的火灾风险。一旦检测到异常情况,系统会立即发出警报,并采取相应的应急措施,如自动切断电源、启动灭火装置等,以防止火灾的发生。
此外,AI火灾检测预警技术还可以通过大数据分析,不断优化和提升其检测能力。通过对历史数据的分析,系统可以学习和识别出更多的火灾风险模式,从而提高预警的准确性和及时性。同时,该技术还可以与其他智能系统进行联动,如与消防系统、监控系统等进行数据共享和协同工作,进一步提升整体的安全管理水平。
总之,AI火灾检测预警技术在电动车充电领域的全面应用,不仅能够有效预防火灾事故的发生,保障用户的生命财产安全,还能提升充电设施的智能化管理水平,推动电动车行业的健康发展。我们将在这篇科普文中介绍关于电能质量分析以及电池火灾成因分析的内容。
随着电动二三轮车(以下简称电动自行车)的普及,其安全性特别是在充电过程中的安全性问题日益突出。锂电池在生产、充电及使用过程中存在火灾风险高、火灾蔓延速度快等问题。AI技术的发展为解决这些问题提供了新的视角。
一、AI技术的重要性
AI技术能够通过大数据分析、机器学习等手段,实现对电动自行车电池状态的精确监测和预测。不仅帮助提前发现电池的异常状态,还能为用户提供个性化的充电建议,从而降低因不当充电操作引发的风险。
二、AI技术的应用场景
- 实时监测预警:AI系统能够持续收集电池的电压、电流、温度等运行数据,并通过学习算法来判断是否存在过热或其他异常情况。一旦检测到潜在风险,系统会立即发送警告信息给用户或监控中心。
- 故障诊断与预测:自适应神经网络算法可以识别电池健康状况的变化趋势,并预测可能发生的故障。例如,电池的老化速度加快、容量下降等现象都可以通过AI系统进行早期识别,从而提醒用户或维修人员及时进行维护保养。
- 电能质量分析:AI技术能够对电动自行车在充电过程中的电能质量进行分析,识别不稳定电源或电网波动对电池的影响,帮助用户避免因电源质量问题导致的电池损坏或故障。
- 智能调度与应急响应:在紧急情况下,AI可以辅助决策,提供最优的应急处置方案。比如,通过分析事故地点周围的资源分布,快速调配最近的救援队伍前往处理。
三、技术实现途径
为了确保电动自行车在充电过程中的安全性,AI技术的应用主要涉及以下几个步骤:
- 数据采集:利用物联网技术,收集电动自行车电池的实时运行数据,包括但不限于电池的温度、电流、电压等信息。
- 数据分析:通过自适应神经网络算法,对收集到的数据进行深度分析,识别出可能导致故障的异常模式。
- 预警机制:基于分析结果,建立预警机制,当检测到电池存在潜在风险时,系统将即时发出警报。
- 用户交互:开发相应的应用程序或平台,使得用户能够接收到预警信息,并获取如何安全充电的操作指南。
- 远程监控:通过云端服务,实现电动自行车电池状态的远程监控,为制造商和服务提供商提供数据支持,便于他们提供更好的售后服务和技术支持。
四、自适应神经网络算法的作用
自适应神经网络算法是一种能够随着时间推移自我调整的机器学习方法,它可以根据不同的输入数据自动调整权重和结构,以提高预测准确性。在电动自行车充电事故检测预警系统中,自适应神经网络算法可以:
- 动态学习:通过对大量历史数据的学习,自适应神经网络能够捕捉电池状态变化的规律,即便是在电池老化或环境条件改变的情况下,也能保持较高的预测准确性。
- 实时更新:随着新数据的不断加入,算法能够实时更新模型,确保其始终处于最佳状态,以应对电池性能的任何变化。
- 复杂关系建模:自适应神经网络擅长处理非线性关系和多变量之间的复杂相互作用,这对于理解电池内部复杂的化学和物理过程至关重要。
五、电能质量分析与电池火灾成因分析
在电动自行车充电过程中,电能质量直接影响着电池的使用寿命和安全性。AI技术可以通过监测电网频率、电压波动等电能质量指标,及时发现不稳定电源或电网波动,避免因此而导致的电池损坏或故障。
此外,AI技术还能通过对电池火灾案例的历史数据进行分析,识别火灾的常见成因,如过度充电、电池短路、电池内部缺陷等。通过这些分析结果,可以进一步优化充电策略,预防火灾事故的发生。
六、未来展望
随着AI技术的不断发展,我们可以预见更多的智能解决方案将应用于电动自行车的安全管理之中。除了上述提到的实时监测预警系统之外,未来的应用场景还包括但不限于:
- 个性化充电策略:基于用户的使用习惯和车辆的实际状态,AI系统可以生成个性化的充电策略,帮助用户延长电池使用寿命。
- 电池健康管理:通过定期的健康检查,AI能够预测电池的剩余寿命,并为用户提供更换或维修建议。
- 环境适应性分析:AI技术还能够考虑外部环境因素对电池的影响,比如气温变化、湿度等,从而为用户提供更为全面的使用建议。
综上所述,AI技术的应用不仅提升了电动自行车充电过程中的安全性,也为整个电动自行车产业带来了新的变革机遇。
No responses yet